Novel clinical phenotypes, drug categorization, and outcome prediction in drug-induced cholestasis: Analysis of a database of 432 patients developed by literature review and machine learning support.
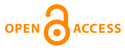
Autors de IIS La Fe
Autors aliens a IIS La Fe
- López-Pascual E
- Rapisarda A
- Drees A
- Steffensen IL
- Luechtefeld T
- Serrano-Candelas E
- de Lomana MG
- Gadaleta D
- Dirven H
- Vinken M
Grups d'Investigació
Abstract
BACKGROUND: Serum transaminases, alkaline phosphatase and bilirubin are common parameters used for DILI diagnosis, classification, and prognosis. However, the relevance of clinical examination, histopathology and drug chemical properties have not been fully investigated. As cholestasis is a frequent and complex DILI manifestation, our goal was to investigate the relevance of clinical features and drug properties to stratify drug-induced cholestasis (DIC) patients, and to develop a prognosis model to identify patients at risk and high-concern drugs. METHODS: DIC-related articles were searched by keywords and Boolean operators in seven databases. Relevant articles were uploaded onto Sysrev, a machine-learning based platform for article review and data extraction. Demographic, clinical, biochemical, and liver histopathological data were collected. Drug properties were obtained from databases or QSAR modelling. Statistical analyses and logistic regressions were performed. RESULTS: Data from 432 DIC patients associated with 52 drugs were collected. Fibrosis strongly associated with fatality, whereas canalicular paucity and ALP associated with chronicity. Drugs causing cholestasis clustered in three major groups. The pure cholestatic pattern divided into two subphenotypes with differences in prognosis, canalicular paucity, fibrosis, ALP and bilirubin. A predictive model of DIC outcome based on non-invasive parameters and drug properties was developed. Results demonstrate that physicochemical (pKa-a) and pharmacokinetic (bioavailability, CYP2C9) attributes impinged on the DIC phenotype and allowed the identification of high-concern drugs. CONCLUSIONS: We identified novel associations among DIC manifestations and disclosed novel DIC subphenotypes with specific clinical and chemical traits. The developed predictive DIC outcome model could facilitate DIC prognosis in clinical practice and drug categorization.
Copyright © 2024 The Authors. Published by Elsevier Masson SAS.. All rights reserved.
Dades de la publicació
- ISSN/ISSNe:
- 0753-3322, 1950-6007
- Tipus:
- Article
- Pàgines:
- 116530-116530
- PubMed:
- 38574623
- Factor d'Impacte:
- 1,194 SCImago ℠
- Quartil:
- Q1 SCImago ℠
BIOMEDICINE & PHARMACOTHERAPY Elsevier Masson
Documents
- No hi ha documents
Filiacions
Keywords
- Drug risk assessment; Drug-induced cholestasis; Machine-learning assisted literature review; Prognosis model; Toxic cholestasis phenotypes
Projectes associats
DESARROLLO DE UN MODELO HEPATOCELULAR HUMANO DIFERENCIADO PARA ESTUDIOS DE METABOLISMO Y POTENCIAL INDUCTOR DE NUEVOS FARMACOS
Investigador Principal: JOSÉ VICENTE CASTELL RIPOLL
SAF2003-09353 . 2003
DEVELOPMENT OF A HIGH THROUGHPUT GENOMICS-BASED TEST FOR ASSESSING GENOTOXIC AND CARCINOGENIC PROPERTIES OF CHEMICAL COMPOUNDS IN VITRO
Investigador Principal: JOSÉ VICENTE CASTELL RIPOLL
CARCINOGENOMICS . COMISION EUROPEA . 2006
LINTOP
Investigador Principal: JOSÉ VICENTE CASTELL RIPOLL
LSHB-CT-2006-037499 - LINTOP . FUNDACIÓN PARA LA INVESTIGACIÓN DEL HOSPITAL UNIVERSITARIO LA FE DE LA COMUNIDAD VALENCIANA . 2005
MECANISMOS TRNACRIPCIONALES IMPLICADOS EN LA ETIOLOGIA DEL HIGADO GRASO NO ALCOHOLICO. ESTUDIOS EN UN MODELO CELULAR HUMANO DE ESTEATOSIS Y APLICACION AL TRANSPLANTE DE HEPATOCITOS EN TERAPIA CELULAR.
Investigador Principal: RAMIRO JOVER ATIENZA
PI07/0550 . INSTITUTO DE SALUD CARLOS III . 2007
MECANISMOS TRANSCRIPCIONALES IMPLICADOS EN EL HIGADO GRASO NO ALCOHOLICO DE ORIGEN METABOLICO E IATROGENICO: INFLUENCIA DE LA RESISTENCIA A LA INSULINA
Investigador Principal: RAMIRO JOVER ATIENZA
PI10/00194 . INSTITUTO DE SALUD CARLOS III . 2010
ESTEATOSIS HEPÁTICA POR MEDICAMENTOS: NUEVOS MECANISMOS Y BIOMARCADORES APLICABLES AL DESARROLLO FARMACÉUTICO Y A UNA TERAPIA MÁS RACIONAL EN PACIENTES CON SÍNDROME METABÓLICO.
Investigador Principal: RAMIRO JOVER ATIENZA
PI13/01470 . INSTITUTO DE SALUD CARLOS III; FUNDACIÓN PARA LA INVESTIGACIÓN DEL HOSPITAL UNIVERSITARIO LA FE DE LA COMUNIDAD VALENCIANA . 2014
Nuevos mecanismos y biomarcadores diagnósticos en la colestasis iatrogénica.
Investigador Principal: RAMIRO JOVER ATIENZA
PI17/01089 . INSTITUTO DE SALUD CARLOS III . 2018
Susceptibility factors and non-invasive biomarkers for liver steatosis induced by valproate in pediatric epileptic patients.
Investigador Principal: RAMIRO JOVER ATIENZA
PI20/00690 . INSTITUTO DE SALUD CARLOS III . 2021
IMPACTO DEL ECMO SOBRE LA FARMACOCINÉTICA DE LA ANIDULAFUNGINA.
Investigador Principal: FRANCISCA PÉREZ ESTEBAN
PFI-ANI-2013-01
Biomarcadores metabolicos y otros factores predictivos del sindrome de obstrucción sinusoidal (SOS) en el transplante de progenitores hematopoyeticos
Investigador Principal: JAIME SANZ CABALLER
PI22/01749 . INSTITUTO DE SALUD CARLOS III . 2023
Biomarcadores metabolómicos y otros factores predictivos del síndrome de obstrucción sinusoidal (SOS) en el trasplante de progenitores hematopoyéticos.
Investigador Principal: JUAN BAUTISTA MONTORO GÓMEZ
TPH-EO-SOS-2022 . 2022
YO INVESTIGO RAMIRO JOVER
Investigador Principal: RAMIRO JOVER ATIENZA
INVEST/2022/76 . CONSELLERIA DE INNOVACIÓN, UNIVERSIDADES, CIENCIA Y SOCIEDAD DIGITAL . 2022
Actividad microbiana y vesículas extracelulares bacterianas implicadas en la aparición de los trastornos del espectro autista en niños prematuros: estudio de seguimiento a dos años
Investigador Principal: ANA CRISTINA GARCÍA BLANCO
CIAICO/2022/233 . CONSELLERIA DE INNOVACIÓN, UNIVERSIDADES, CIENCIA Y SOCIEDAD DIGITAL . 2023