Visual interpretability of image-based classification models by generative latent space disentanglement applied to in vitro fertilization
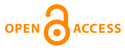
Autores de IIS La Fe
Participantes ajenos a IIS La Fe
- Rotem, Oded
- Schwartz, Tamar
- Maor, Ron
- Tauber, Yishay
- Shapiro, Maya Tsarfati
- Gilboa, Daniella
- Seidman, Daniel S.
- Zaritsky, Assaf
Grupos
Abstract
The success of deep learning in identifying complex patterns exceeding human intuition comes at the cost of interpretability. Non-linear entanglement of image features makes deep learning a "black box" lacking human meaningful explanations for the models' decision. We present DISCOVER, a generative model designed to discover the underlying visual properties driving image-based classification models. DISCOVER learns disentangled latent representations, where each latent feature encodes a unique classification-driving visual property. This design enables "human-in-the-loop" interpretation by generating disentangled exaggerated counterfactual explanations. We apply DISCOVER to interpret classification of in vitro fertilization embryo morphology quality. We quantitatively and systematically confirm the interpretation of known embryo properties, discover properties without previous explicit measurements, and quantitatively determine and empirically verify the classification decision of specific embryo instances. We show that DISCOVER provides human-interpretable understanding of "black box" classification models, proposes hypotheses to decipher underlying biomedical mechanisms, and provides transparency for the classification of individual predictions. Identifying complex patterns through deep learning often comes at the cost of interpretability. Focusing on the interpretation of classification of in vitro fertilization embryos, the authors present DISCOVER, an approach that enables visual interpretability of image-based classification models.
Datos de la publicación
- ISSN/ISSNe:
- 2041-1723, 2041-1723
- Tipo:
- Article
- Páginas:
- -
- Factor de Impacto:
- 4,846 SCImago ℠
- Cuartil:
- Q1 SCImago ℠
NATURE COMMUNICATIONS NATURE PUBLISHING GROUP
Documentos
- No hay documentos
Filiaciones
Filiaciones no disponibles
Proyectos asociados
Estudio del efecto del estrés en la ventana de implantación y su influencia en el éxito reproductivo
Investigador Principal: PATRICIA DÍAZ GIMENO
FPU19/03247 . MINISTERIO DE CIENCIA E INNOVACION . 2020
Desarrollo de modelos de selección de embriones basados en inteligencia artificial para predecir las condiciones ideales que mejoren la probabilidad de éxito de un tratamiento de reproducción asistida.
Investigador Principal: MARCOS MESEGUER ESCRIVÁ
PI21/00283 . INSTITUTO DE SALUD CARLOS III . 2022
Efecto de la morfología y morfocinética del blastocisto biopsiado en la supervivencia y resultados clínicos después del procedimiento de vitrificación-desvitrificación.
Investigador Principal: MARCOS MESEGUER ESCRIVÁ
FPU20/03621 . MINISTERIO DE CIENCIA E INNOVACION; MINISTERIO DE UNIVERSIDADES . 2021
Desarrollo e implementación de un algoritmo basado en inteligencia artificial para la selección de embriones desvitrificados a partir de datos morfológicos, morfocinéticos y secretómicos
Investigador Principal: MARCOS MESEGUER ESCRIVÁ
CIACIF/2021/019 . CONSELLERIA DE INNOVACIÓN, UNIVERSIDADES, CIENCIA Y SOCIEDAD DIGITAL . 2022
Desarrollo funcional y clínico del algoritmo de Inteligencia Artificial SSE (Software para la Selección de Espermatozoides basado en IA) en tratamientos de fecundación in vitro (FIV).
Investigador Principal: MARCOS MESEGUER ESCRIVÁ
CIACIF/2022/438 . CONSELLERIA DE INNOVACIÓN, UNIVERSIDADES, CIENCIA Y SOCIEDAD DIGITAL . 2023
Cita
Rotem O,Schwartz T,Maor R,Tauber Y,Shapiro MT,Meseguer M,Gilboa D,Seidman DS,Zaritsky A. Visual interpretability of image-based classification models by generative latent space disentanglement applied to in vitro fertilization. Nat Commun. 2024. 15. (1):7390. IF:14,700. (1).